tl;dr: I made ViHN to read Hacker News without having to move my hands away from the keyboard. It’s freely available on Firefox and Chrome.
This is about yet another Hacker News extension. But other people with my obsession for killing the mouse might enjoy it.
Most of the content of this article is just an ancient1 talking about the good ol’ times. But you can jump directly to the description of the extension.
btw, I use Vim
I know that I am not the only one who had a keyboard-zealot phase.
When GNOME Shell happened, I was forced to go looking for a new window manager. I ended up installing Ratpoison. Like the more well-known i3, Ratpoison is a tiling window.
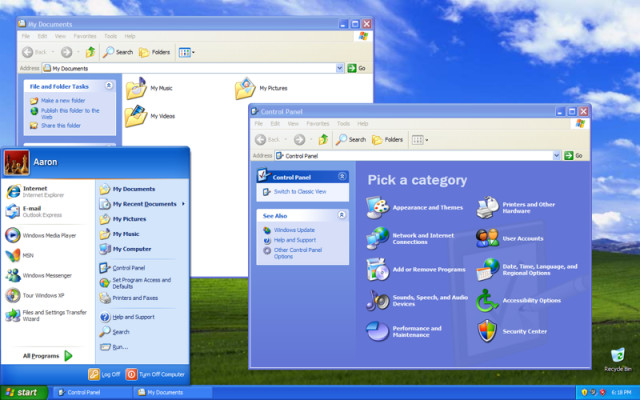
That means that, instead of floating windows you usually move around with the mouse, you split the screen in “tiles” however you like, and assign windows to them. By default, the screen is made of a single tile, so windows are full-screen. Then, you might split it in two halves, to show two windows side-by-side (for instance, a text editor and a Wikipedia page). It might seem pointless since you can also do this with regular (“stacking”) window manager, but the important difference is the workflow.
With a regular window manager, positioning windows can feel somewhat limited. As a beginner, you might use the mouse to arrange some windows so that they display all the information you need in a convenient way. But that takes several seconds. And people like me do not have seconds to waste — we could be using it to read memes on Reddit instead.
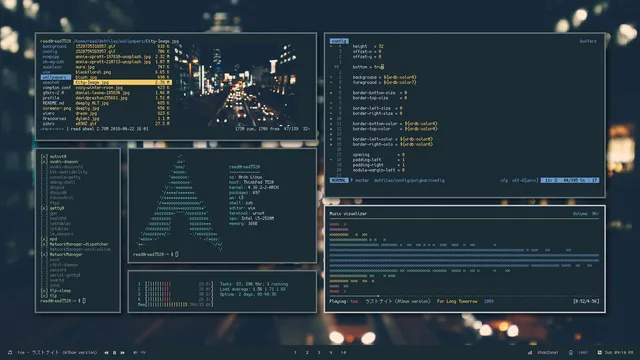
So we learn the keyboard shortcuts to arrange the current window in the left/right half of the screen. Unfortunately, there are times when you would like to show three windows at the same time. And then, you have to go back to using the mouse. Can you imagine the horror?
With a tiling window manager, we can use keyboard shortcuts to display how many windows in however way we want. Want to display 81 different windows in a 9×9 grid to make pixel art maximize your productivity? Of course, just a few keystrokes.
The real benefit, however, is that no one else will be able to use your computer. You will have switched to another tiling window manager by the time they figure out the previous one.
Note: However, Ratpoison is a misnomer: since you can still use your mouse to open links in Firefox instead of Tab-Tab-Tab-Tab-Tab-Tab-Tab-Tab-Tab-Tab-Tab-Tab-Return like a normal person2. Because of Valve, you even have to click on terrorists, instead of just spotting the “g” in the middle of random punctuation. Thankfully, you can live in the TTY, read documents with fbi and watch videos with libcaca.
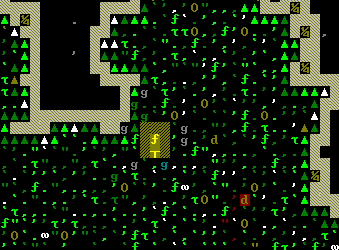
Since, I have strayed from the rightful path, and switched to xfce43. I even tried Visual Code for a while (with Vim key bindings, of course), but I always come back to Vim.
Hacker News
When I was younger, and more innocent, I used to kill time by looking for script kiddies hideouts with advanced search keywords such as “hacker forum”. I would enjoy watching various people trying to impress each other with technical nonsense.
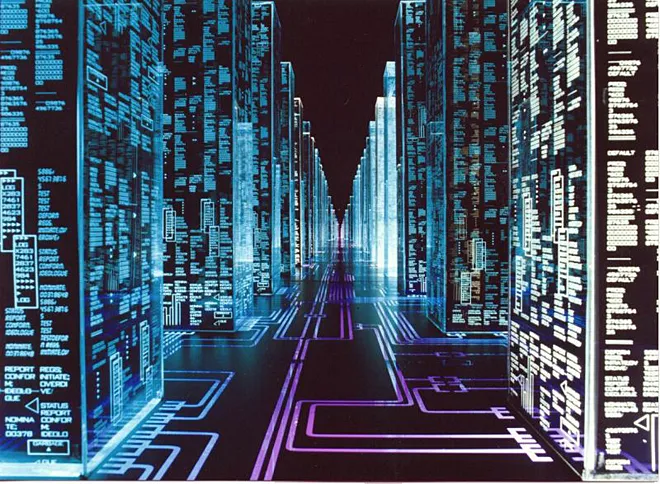
At some point, I stumbled upon Hacker News, which is actually not a hideout for script kiddies5. It’s actually a place to promote great business ideas, discuss major security flaws underpinning infrastructure of geopolitical importance, and explore advanced technical topics. And not even half the content is about the latest technological fad6.
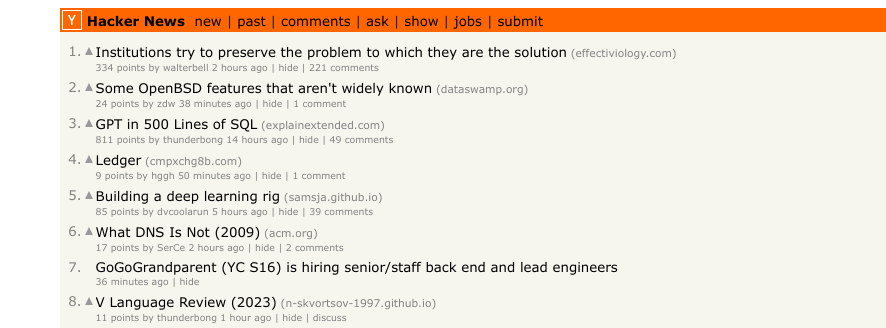
Unfortunately, it uses that quirky system where the discussion is organized in trees, and people vote for the most interesting comments. In older forums, you simply had to read the 3000-message-long thread to find the few insightful remarks. And with 20 messages per page, that would be barely 150 pages to read!
These fancy vote-based forums allow you to skip entire side-discussions of tangentially related concepts. It’s such a shame that you won’t have to learn about the proper way to repair drywall in an American house when you were just curious about the newest JavaScript framework.
In any case, the point is that reading a comment tree involves more than just scrolling done in a linear thread. Instead, you might want to go back up to the parent, skip to the next sibling discussion, hide a sub-tree, upvote or reply to a specific comment.
And as discussed above, I am not going to do all that hard work with a mouse pointer.
Procrastinating More Efficiently
At first, I just wrote myself some user script to use J, K, and other keys to navigate Hacker News comments, after having done something similar for SlateStarCodex. That was mostly good enough, but I was not as systematic with code versioning at the time, and the format did not particularly encourage it. So I eventually lost that script.
This led me to Refined Hacker News, which is actually pretty good. Unfortunately, some things are broken, and it can take a while to load. And since it is unmaintained, I did not have any hope of being able to fix the most annoying issue (the extension sometimes not working at all).
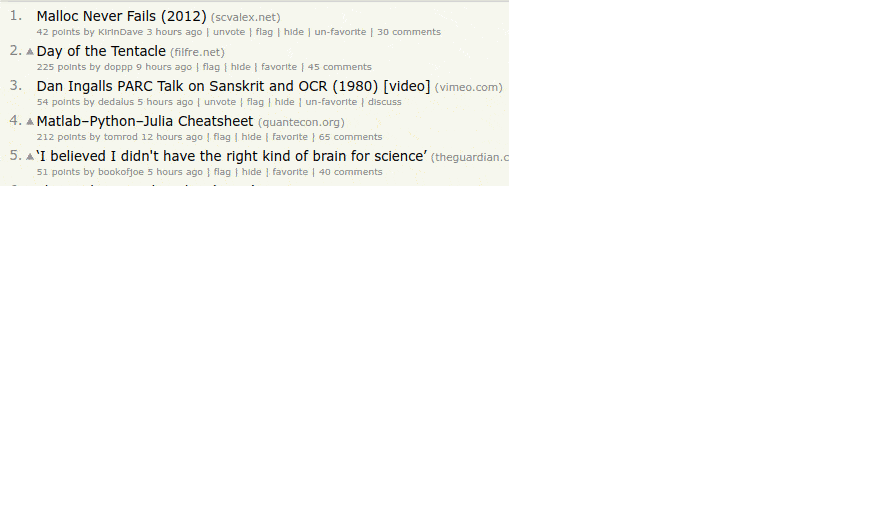
So, I finally made my own browser extension for Firefox and Chrome. It’s called “ViHN”. “Vi” as a shorthand for Vim7, and “HN” for “Hacker News”. Get it? I know, it’s very subtle.
It is primarily focused on keyboard-based navigation, should introduce no visible delay, and minimize surprises. Of course, it has many Vim-like key bindings to navigate comments (not just J and K). You can look at the README for the list, but I have also included it below to save you a mouse click if you are not using Vimium.
Also, I stole the idea from bakkot’s SlateStarComments to quickly find new comments. I never quite liked the idea of trying to remember what comments the user might have seen. This is easily confounded by reloading the page by mistake, not having the time to read everything at that particular time, not using the same browser or computer, and so on. Instead, ViHN shows a list of comments in reverse chronological order. You can go through that list (of course using key bindings), and stop when you get to those you already read.
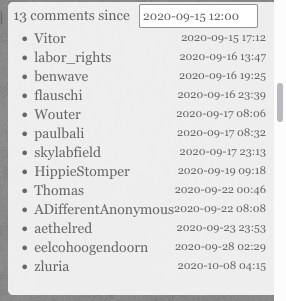
Among other features, you can quick-reply/edit/delete comments, preview comments when replying/editing, it highlights op.
A less visible feature is that manages requests (votes, favorite, flag, persistent collapse) and spread them in time to avoid the drastic rate limiting of Hacker News. If a request results in a 503 error (non-standard “too many requests”), ViHN will retry it later. This materializes in the form of “…” instead of “favorite”/“flag” in links and spinning arrow for votes8.
But this would not be worthy of Hacker News if this was not used as an occasion to bike-shed a particular JavaScript framework. The extension is written is Vanilla JS. This enables maximal performance with minimum overhead and zero build time.
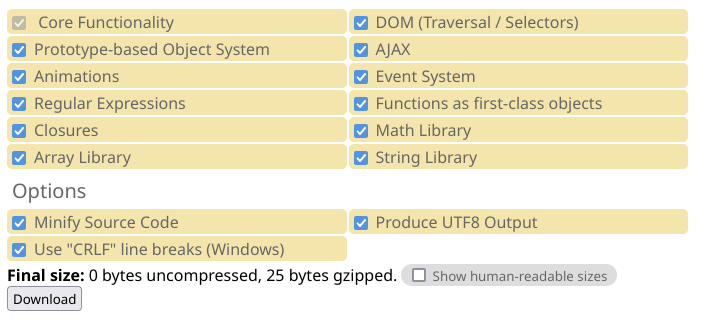
Key bindings
This section is reproduced from the README, and also available in the extension by pressing ? to toggle help.
Navigate Comments/Stories
Key | Effect |
---|---|
j | Next comment/story |
k | Previous comment/story |
J | Next sibling comment |
K | Previous sibling comment |
g | Go to first story/comment |
G | Go to last story, last root comment or “More” link |
H | Focus on story/comment at the top of the screen (high) |
M | Focus on story/comment in the middle of the screen |
L | Focus on story/comment at the bottom of the screen (low) |
n | Switch to Newest Items |
h | Parent comment/story (see [#follow-links](Follow Links)) |
p | Parent comment/story (see [#follow-links](Follow Links)) |
Note: You can also select an item by clicking in its bounding box.
Follow links
Key | Effect |
---|---|
o | Open story link/comment |
O | Open story link/comment in background |
c | Open comment thread |
C | Open comment thread in background |
b | Open both story link and comment thread |
B | Open both story link and comment thread in background |
h | Follow “context” link (go to comment thread, but focus on current comment) |
p | Follow “parent” link (go to parent’s page, and focus on parent comment/story) |
1 | Open 1st link in comment (maintain shift to open in background) |
… | … |
9 | Open 9th link in comment (maintain shift to open in background) |
0 | Open 10th link in comment (maintain shift to open in background) |
Note: When on the “XXX more comments” link, you can hit either of [lLcC]
to go to the next page of comments.
Note: The digits of the numeric keypad work as well to open links in comments. However, this can only open links in foreground.
Note: When using AZERTY, the key bindings to open links inside comments still work like in QWERTY. Hit the 1 key without shift (like typing &
) to open the 1st link in foreground. Hit the 1 key with shift (like typing 1
) to open the 1st link in background. Same for the other link numbers.
Actions
Key | Effect |
---|---|
m | Collapse/uncollapse comment tree |
u | Upvote story/comment, or cancel vote |
d | Downvote story/comment, or cancel vote |
f | Favorite/un-favorite story/comment of the current page |
F | Flag/unflag story/comment of the current page |
r | Comment on story, or reply to comment (with preview) |
e | Edit comment (with preview) |
D | Delete comment |
Ctrl+Return | Submit current form |
Navigate Newest Items
In the Newest Items list, the following key bindings are available:
Key | Effect |
---|---|
l | Show selected comment/story |
j | Next comment/story |
k | Previous comment/story |
J | Jump 10 down |
K | Jump 10 up |
g | Go to top story/comment |
G | Go to last story/comment |
n | Switch back from Newest Items |
- I mean, I was born in the previous millenium! ↩︎
- Of course, you could just use Vimium, but where is the fun in that? ↩︎
- While writing this article, I learned that Linus Torvalds also switched to Xfce4 at this time. So, maybe, I am not such a bad person. ↩︎
- No, it wasn’t. The still is from the marvelous Hackers movie. But we did have Packard Bell Navigator, which was just as good (no, it wasn’t). ↩︎
- Or maybe it is, and I have become one without even realizing. ↩︎
- I like making fun of Hacker News, but is genuinely pretty good place to keep oneself informed, when you are into computers. If you want some more fun, read every Hacker News thread ever. ↩︎
- I only ever use Vim in practice, never vi, never ed, so I like to pretend it’s all the same. ↩︎
- There is no visible feedback for collapsing since that happens immediately, and the request can be handled asynchroneously. ↩︎